What It Is & How It Works
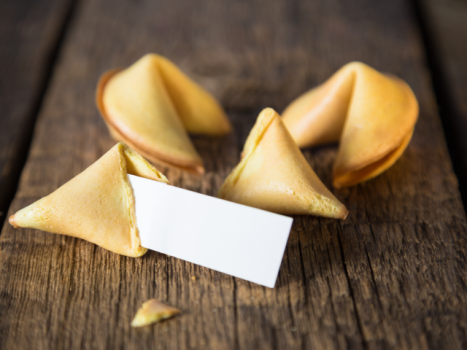
[ad_1]
The Gist
- Predictive analytics definition. This advanced analytics branch uses statistical algorithms and machine learning to predict future events.
- Predictive’s role. Healthcare, marketing and insurance are just a few of the industries utilizing predictive analytics.
- Challenge’s ahead. Data quality and avoiding over-reliance on predictions are key hurdles to effective use of predictive models.
Editor’s Note: This article has been updated on October 26, 2023 to include new data and information; the original content was authored by Lesley Harrison.
In the world of data science, predictive analytics is a crucial component in the toolkit of modern businesses. And as companies work through the complexities of the ever-evolving digital age, understanding the key differences between types of data analytics is paramount. There are four major types of data analytics that organizations turn to: descriptive, diagnostic, predictive and prescriptive.
- Descriptive Analytics: Answers the question, “What happened?”
- Diagnostic Analytics: Answers the question, “Why did it happen?”
- Predictive Analytics: Answers the question, “What could happen?”
- Prescriptive Analytics: Answers the question, “What should you do next?”
Our focus today is on how predictive analytics operates, delving into its intricacies and looking at how businesses can harness its potential.
What Is Predictive Analytics?
To provide a predictive analytics definition, it’s a branch of advanced analytics using statistical algorithms and machine learning techniques to identify the probability of future events based on historical data. It also utilizes tools and techniques like data mining, modeling and artificial intelligence, all with the goal of analyzing data, identifying patterns and making predictions. Organizations that use this form of data analysis can anticipate future outcomes, identify risk and are better positioned to make decisions.
Related Article: Exploring Customer Data: Definition, Types & Usage
The History of Predictive Analytics
Predictive analytics can be traced back to early statistical models and data mining techniques, which laid the groundwork for modern data-driven decision-making. Over the years, as computational capacities expanded, predictive analysis evolved from simple linear regressions to sophisticated algorithms under the umbrella of machine learning.
The convergence of vast datasets, enhanced computing power and innovative algorithms propelled predictive analytics to the front of business leaders’ attention, allowing organizations to forecast future outcomes with high accuracy. As these methodologies matured, predictive analytics transformed from a niche specialty to a mainstream tool, assisting industries from healthcare to finance.
Predictive Analytics vs. Prescriptive Analytics
Predictive and prescriptive analytics both serve distinct roles in the world of data-backed decision-making. While predictive analytics uses past data to forecast future outcomes, prescriptive analysis goes a step further, offering actionable recommendations on what to do next based on those predictions.
5 Examples of Predictive Analytics in Action
Predictive analytics techniques can be used to tweak and test processes across a variety of industries. Earl Sires, senior product marketer at EAB and previously digital content marketer at Rapid Insight explained, “Many industries use predictive analytics as a core part of their strategy.”
Some common use cases include:
- Healthcare
- Marketing
- Insurance
- Supply chain management
- Fund management
1. Predictive Analytics in Healthcare
Healthcare workers use predictive analytics in a variety of ways to improve the efficiency of the service they provide. Effective modeling of patient data can also help improve patient outcomes. “Outside factors, known as Social Determinants, can play a greater role in your patient’s health than anything that happens within the hospital doors,” said Sires.
2. Predictive Analytics in Marketing
Marketing is an industry that relies heavily on metrics. Marketers track clicks, engagement, views and other behaviors. Brands can use predictive analytics to take a huge database of information and score leads based on how likely they are to buy a product. This gives brands an idea of where they should prioritize their outreach to get the biggest return on investment.
3. Predictive Analytics in Insurance
The insurance industry, like the marketing industry, is driven by statistics. Accident reports and historical data are used to judge the risk factors for individual clients. Predictive analytics can help with processing claims and preventing fraud.
Jason Rodriguez of Instant Insight said predictive analytics could reduce the need for professional oversight in areas, such as loss handling and initial triage. Fraud is a huge issue for insurance companies, and models that highlight suspected fraud could save time and money for underwriters.
4. Predictive Analytics in Supply Chain Management
Sires explained that companies can use predictive analytics to “model different risk factors to see how they impact your supply chain and incorporate information from disparate sites or sources into one model to get the most accurate, relevant picture of your operation.”
The information from a predictive model can then be used to prioritize shipments or guide the creation of prescriptive models. Supply chains can be complex and have many points of failure. By examining each of these points in turn, organizations can make their supply chains more robust and adaptable.
5. Predictive Analytics in Fund Management
Deloitte looked at a major financial organization that transformed from being a risk-averse pension fund into a risk management organization. The company’s old systems weren’t capable of the more complex models required for new investments. Using predictive project analytics (PPA), the company was able to run models to determine whether the safest course of action was to update the systems all at once or step-by-step. By following the suggestions given by predictive modeling, the company completed its update ahead of schedule and under budget.
Related Article: How Can Predictive Analytics Impact Customer Experience?
Where Does Predictive Analytics Work Best?
Predictive analytics work best in scenarios where forecasting is important, especially for short- and medium-term trends. From assessing market trends to gauging customer behaviors, it’s useful in areas that demand proactive (rather than reactive) strategies. Some examples of when to apply predictive analytics include:
- Product demand
- Pricing strategies
- Revenue forecasting
- Customer retention
- Maintenance scheduling
- Risk assessment
- Talent acquisition
Prescriptive analytics models are more complex to build, but they allow an organization to explore multiple what-if scenarios. Meanwhile, predictive analytics models focus on a more narrow set of parameters. This means these models are easier to build and can provide a quick overview of a situation.
Predictive analytics helps bring clarity and objectivity to decision-making. It can inform major spending or policy decisions in situations where managers may otherwise be prone to wishful thinking. Models cannot predict the future with 100% accuracy, but they can assist with making educated guesses.
How Does Predictive Analytics Work?
Predictive models can have varying degrees of complexity, but the principle remains the same. They use known results to develop models (or train AI) to predict future values for different or new data. Predictive analytics models provide predictions that represent the probability of a target variable (such as profit or customer churn) based on the estimated significance from the set of input variables.
Three common predictive modeling techniques include decision trees, regression and neural networks.
Decision Trees
Decision trees partition data into subsets. Each subset is based on categories of input variables. A decision tree has branches representing different choices and leaves representing classifications or decisions.
Decision trees are a popular choice to predict future behavior because they’re easy to understand and provide a visual representation of the information. In addition, they handle missing values well, meaning they’re useful for building quick, simple models when you don’t have all the information required for a more complex model.
Regression
The two types of regression analysis used in predictive analytics are linear regression and logistic regression. These types of analysis are useful for estimating the relationships among variables. Regression models are intended for use on continuous data, especially if that data can be estimated to follow a normal distribution.
One common application of regression models is ecommerce predictions, such as predicting how product prices might affect sales. More sophisticated models, such as multiple regression, can model the outcome of situations that have multiple variables.
Neural Networks
Models that are incredibly complex and have multiple variables lend themselves to the application of neural networks. These models can handle nonlinear relationships in data. Neural networks use pattern recognition and may also apply some AI to model the parameters. For a neural network to be effective, it will most likely require a significant amount of training data.
Related Article: Predictive Analytics: Overcoming Data Swamps in Tech’s Dynamic Landscape
The Benefits of Predictive Analytics
Predictive analytics plays a part in shaping today’s business strategies. Let’s take a look at some of the core advantages to get a better understanding of the technology’s real-world impact.
Informed Decision-Making
Predictive analysis dives deep into data to unveil patterns and trends, letting businesses make decisions grounded in evidence. It’s also useful for scenarios where a business needs to make predictions about outcomes but doesn’t have a lot of information available. For example, a retailer might use predictive analytics insights to determine which products will most likely become popular in the upcoming holiday season and stock accordingly.
Operational Efficiency & Cost Reduction
Predictive modeling helps organizations optimize processes, reduce waste and increase productivity, all of which lead to reduced costs. An airline, for example, might use predictive tools to anticipate maintenance needs, minimizing downtime and ensuring smoother operations.
Improved Customer Experience
By understanding and anticipating the future behavior of consumers, businesses can tailor their offerings to provide more relevant and personalized customer experiences. Amazon’s recommendation engine, for example, suggests products based on users’ browsing and purchasing histories.
Risk Management
Predictive analytics can identify potential risks and vulnerabilities, allowing companies to implement preventive measures before issues arise. Financial institutions, for example, use predictive analytics tools to forecast loan defaults, making sure they maintain a healthy portfolio.
The Challenges of Predictive Analytics
While predictive analytics offers a number of benefits in understanding and anticipating trends, it’s not without its challenges.
Data Quality & Integrity
For predictive analytics to be effective, it requires high-quality data. However, data mining from varied sources often brings in inconsistencies, noise and errors. For example, a healthcare company might find discrepancies in patient records, leading to misleading analysis if not cleaned and processed.
Complexity & Expertise Required
Using predictive models requires a deep understanding of statistical techniques and the nuances of the specific sector or problem. Without the right expertise, there’s a risk of misinterpreting results. Businesses often need to invest in a talented team of data scientists to fully leverage these tools.
Over-Reliance on Predictions
Predictive analytics offers a lot of valuable, actionable insights. But at the end of the day, they’re still predictions. Over-relying on them without considering external factors or using human judgement can lead to overconfidence and potentially faulty decisions. It’s important to strike a balance between data-backed insights and real-world considerations.
Related Article: Get Rid of Garbage Data: 4 Ways to Ensure Quality Data
The Future of Data Analytics
Data is more readily available than ever before, and businesses can take advantage of predictive models to turn that data into actionable insights. Experts predict the predictive analytics market will increase at a rapid rate, growing from $5.29 billion in value in 2020 to an estimated $41.52 billion by 2028.
Those who want to learn more beyond the predictive analytics definition and use cases should further explore the areas of machine learning, big data and artificial intelligence. Data analysis is a field offering lifelong learning opportunities and new challenges.
[ad_2]
Source link