The Future of CX Personalization: AI and Data Analytics
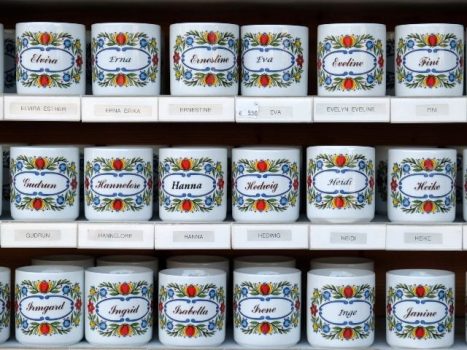
[ad_1]
The Gist
- Data harnessing. AI analytics empowers businesses to use data effectively in CX.
- Enhanced personalization. AI tools deliver personalized experiences, boosting customer retention.
- Operational efficiency. Automation in AI analytics streamlines processes and saves valuable time.
Customer experience (CX) professionals say they and their clients are using artificial intelligence (AI) analytics to harness data and drive customer personalization in several key areas: from customer friction to churn, sentiment and engagement. CX executives in various industries — such as software, marketing and finance — are seeing multiple trends in how customer data and AI analytics are being combined to improve overall CX. A group of CX, data and AI execs shared details on those trends with CMSWire:
1. Cutting Customer Churn and Increasing Retention
Cristina Fonseca, head of AI at San Francisco-based Zendesk, a maker of CX software, said two-thirds of consumers are “willing to share additional data to enable AI-driven personalized experiences,” according to the Zendesk “CX Trends Report.”
Fonseca said the finding presents companies with an “unprecedented chance to cultivate stronger relationships and enhance customer retention.”
For instance, Zendesk is training its AI capabilities on its large CX data sets to “better understand consumers” and help customer service agents “increase their knowledge and provide personalized experiences,” Fonseca said.
AI analytics models built on these types of customer profile information, transactional data and external data are predicting customer churn as well as upsell opportunities, said Zohar Bronfman, co-founder and CEO of Pecan AI , an automated predictive analytics platform based in Ramat Gan, Israel.
“In both of these cases, AI can anticipate customer behaviors in advance,” Bronfman said.
Predictive AI analytics give companies “a heads-up about what customers are likely to do, so they can take personalized action in advance,” Bronfman said.
Monica Ho, chief marketing officer at San Diego-based SOCi, a marketing platform for multilocation brands, said 92% of customer questions posted on retailer Google profiles go unanswered, and marketers “ghosting” customers online costs retailers $2.4 billion annually, according to the SOCi “Local Visibility Index.”
Ho said that with data on customer inquiries, AI chatbots efficiently handle customer questions and provide real-time responses, “ensuring that no customer is left waiting or feeling ignored.”
Marketers apply AI analytics in the customer service case to enhance “brand reputation, deliver exceptional customer experiences, and foster long-term loyalty,” Ho said.
Related Article: The 5 Stages of Predictive Analytics for CX Success
2. Improving Customer Insights With Sentiment Analysis
Fonseca with Zendesk said companies are using AI analytics to drive “business value” through “intent detection and sentiment analysis for customer personalization.”
When AI analysis, for instance, detects a customer’s “specific intent” is to make a return, their inquiry can get “instantly routed to the correct customer service representative instead of routing them through a generic queue,” Fonseca said.
Jonathan Moran, head of martech marketing at Cary, North Carolina-based SAS, a maker of analytics software, said text analytics and sentiment analysis are “a must-have for conversational AI.”
“By analyzing chat text strings and the sentiment in those text strings, brands can understand customer attitudes and intent,” Moran said.
Companies are training their AI chatbots to “analyze the text from customer interactions, understand the sentiments, identify common product inquiries, and even predict possible issues before they arise,” said Tim Shi, co-founder and chief technology officer of Palo Alto, California-based Cresta, a maker of generative AI for contact centers.
Shi said CX professionals are using AI analytics to “track and interpret customer behavior and sentiment trends over long periods.”
“This capability provides an understanding of the customer’s journey and allows businesses to identify and respond to any anomalies or sudden changes in these trends,” Shi said.
AI analytics enable CX teams to analyze customer feedback, reviews and survey responses to extract valuable insights on sentiment and preferences, said Rhodette Zuñiga, VP of customer success at Seattle-based Textio, a maker of HR software.
Zuñiga said that with AI sentiment analysis, CX teams “aggregate and assess customer feedback across various channels, effectively identifying common pain points and areas for improvement.”
Related Article: Sentiment Analysis Improves the Customer Experience
3. Reducing Customer Friction
Frank Schneider, VP and AI evangelist at Melville, New York-based Verint, a customer engagement platform, said brands integrating customer data and conversational AI are “less interested in preventing a human contact and more interested in reducing friction at the customer outreach moment of truth.”
CX teams employing conversational AI analytics are “at the forefront of the conversational economy’s ability to think well beyond the archaic metrics of contain and deflect,” Schneider said.
Schneider said conversational AI is opening “front doors across all user experiences and touch points,” such as asynchronous digital messaging and self-service task completion.
“This creates an end-to-end life cycle of conversational access to analytics for CX leaders, customized workflows for contact center agents, and personalized customer journeys,” Schneider said.
For example, conversational AI data is being used, Schneider said, for channel switching, such as a follow-up SMS message, to deliver an “elegant experience” that creates both revenue and contact center efficiency.
Ranjitha Kumar, chief scientist at San Francisco-based UserTesting, a provider of user experience insights, said companies are employing AI to analyze clickstream data to “identify the parts of an interaction where a user struggles or experiences friction — where someone gets stuck or has to try several times to make progress.”
Kumar said, for instance, if a company launched a new product that didn’t reach sales targets, “these sorts of models can help you quickly understand whether people were confused by your checkout flow or if they just thought the product was too expensive.”
“And once you’ve built these building blocks, it’s easy to scale them,” Kumar said. “All of a sudden, it becomes feasible to do UX testing with hundreds of users instead of just a handful.”
4. Optimizing Engagement Throughout the Customer Journey
Sisi Zhang, executive vice president of data science and analytics at the New York-based marketing agency Razorfish, said AI analytics is optimally being used by companies to “provide the insights around consumer journeys to then determine the right activation lever for personalization.”
“To do this, we need more thoughtful design around propensity models that show a consumer’s true propensity to engage or purchase a product,” Zhang said.
“If someone is just browsing, it doesn’t make sense to try to push a purchase event immediately since that is disruptive to the consumer’s experience. However, if someone is close to purchase, and we have clearly identified what signals constitute being further along in the consumer journey, then we would want to serve a different experience to help meet that need.”
Zhang said, for instance, AI analytics are being used by companies to personalize web UX for the most engagement, better match supply and demand for products and model scores for “customer propensity to purchase certain products.”
Sara Varni, chief marketing officer at New York-based Attentive, an SMS marketing platform, said that as brands are taking a “direct approach to data collection, explicitly asking customers to share their product preferences and interests,” and using data from web interactions, companies can “get granular with personalization,” such as sending VIP deals.
However, Varni said, parsing through “massive data sets can take valuable time and bandwidth.”
Using AI analytics tools helps companies “automate time-consuming tasks, such as identifying and planning the highest performing messages, helping teams save time to focus on strategic outcomes that impact the bottom line,” Varni said.
Deven Lindemann, chief customer success officer at Minneapolis, Minnesota-based Datasite, an M&A platform, said it sees the first step in applying AI in customer service as training the AI to “ensure accurate results,” including the history of customer engagements and team knowledge.
Lindemann notes that the company’s next phase of AI productivity tools and insights will focus on personalization, using tone and presentation to make engagement “more conversational and relatable and moving from reactive to proactive engagement.”
5. Unifying Omnichannel CX Data
At SOCi, Ho is seeing AI analytics and automation blend to help marketers “manage, make sense of, and act on data” from across a fragmented ecosystem, streamlining time-consuming tasks.
“Software should do this work for us, and now, with generative AI, it can do it exceptionally well with a high degree of sophistication,” Ho said.
Schneider at Verint said customer service leaders are employing an AI orchestration layer to present smooth handoffs across different channels and technology stacks, due to conversational AI’s “swift recognition of anticipated journeys and easy retrieval and delivery of solutions or pivots via APIs.”
Schneider added that enterprises are now “flush with fuel but in need of a way to mine it in a meaningful way that is personalized to customer persona and profile.”
Generative AI is allowing organizations to “begin to tap into data like never before,” such as generating highly granular dashboards, Schneider said.
“Now you can tell the AI precisely what you want and access timely, rich data that you can act on immediately,” Schneider said.
Ho stressed that if companies now “expect marketers to act as data scientists, that’s a fairly material mindset shift.”
The change is “going to take some time,” and automation is going to be “invaluable” in the process, Ho said.
[ad_2]
Source link