AI’s Game Changers in Customer Interaction
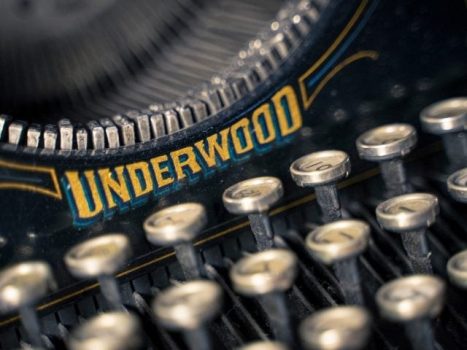
[ad_1]
The Gist
- Revolutionizing communication. NLU and NLP are transforming business-audience communication by enabling unprecedented personalization and relevance in marketing messages.
- Enhancing customer experience. These AI technologies are redefining marketing, improving efficiency in consumer interactions, and offering more engaging and seamless customer experiences.
- Global outreach and understanding. NLU and NLP break language barriers, allowing brands to connect with a global audience while maintaining cultural sensitivity and relevance.
Natural Language Understanding (NLU) and Natural Language Processing (NLP) are pioneering the use of artificial intelligence (AI) in transforming business-audience communication. These advanced AI technologies are reshaping the rules of engagement, enabling marketers to create messages with unprecedented personalization and relevance. This article will examine the intricacies of NLU and NLP, exploring their role in redefining marketing and enhancing the customer experience.
Introduction to NLU and NLP
NLU and NLP have greatly impacted the way businesses interpret and use human language, enabling a deeper connection between consumers and businesses. By parsing and understanding the nuances of human language, NLU and NLP enable the automation of complex interactions and the extraction of valuable insights from vast amounts of unstructured text data. These technologies have continued to evolve and improve with the advancements in AI, and have become industries in and of themselves. According to projections from Fortune Business Insights, the global NLP market is expected to grow from $24.10 billion in 2023 to $112.28 billion by 2030, while the NLU market, which is also experiencing rapid growth, is expected to grow to $35 billion by 2025.
Nikola Mrkšic, CEO and co-founder at PolyAI, a provider of AI-based voice assistants for call centers, told CMSWire that the Natural Language Understanding models that power conversational AI technologies are sophisticated enough to glean the intent of a customer’s conversation rather than just try to identify keywords in what was said like less-capable instances of automated support. “In addition to understanding what the conversation was actually about, NLU models enable virtual agents across digital channels to engage with a customer in language that is natural enough to be mistaken for a human agent.”
Mrkšic related that as callers start to experience what’s possible with NLU/NLP, the conditioned and well-recognized norm of saying “live agent” or smashing zero over and over to try and bypass automated support will fade into history, resulting in an exceptional customer experience from a scalable business process.
The promise of NLU and NLP extends beyond mere automation; it opens the door to unprecedented levels of personalization and customer engagement. These technologies empower marketers to tailor content, offers, and experiences to individual preferences and behaviors, cutting through the typical noise of online marketing. By analyzing customer feedback, social media discourse, and other digital communications, NLU and NLP provide the tools needed to draft messages that resonate on a personal level, creating a sense of understanding and intimacy with a brand.
Additionally, NLU and NLP are pivotal in the creation of conversational interfaces that offer intuitive and seamless interactions, whether through chatbots, virtual assistants, or other digital touchpoints. This enhances the customer experience, making every interaction more engaging and efficient. The integration of NLU and NLP in marketing and advertising strategies holds the potential to transform customer relationships, driving loyalty and satisfaction through a deeper understanding and anticipation of consumer needs and desires.
Related Article: Natural Language Processing and Conversational AI in the Call Center
Foundation of NLU and NLP
NLP and NLU are closely related fields within AI that focus on the interaction between computers and human languages. NLP is the broader discipline that encompasses techniques for processing and analyzing large volumes of natural language data, with the objective of enabling machines to understand and interpret human language in a way that is both meaningful and useful. It includes tasks such as speech recognition, language translation, and sentiment analysis. NLP serves as the foundation that enables machines to handle the intricacies of human language, converting text into structured data that can be analyzed and acted upon.
NLU, a subset of NLP, delves deeper into the comprehension aspect, focusing specifically on the machine’s ability to understand the intent and meaning behind the text. While NLP breaks down the language into manageable pieces for analysis, NLU interprets the nuances, ambiguities, and contextual cues of the language to grasp the full meaning of the text. It’s the difference between recognizing the words in a sentence and understanding the sentence’s sentiment, purpose, or request. NLU enables more sophisticated interactions between humans and machines, such as accurately answering questions, participating in conversations, and making informed decisions based on the understood intent.
The history of NLU and NLP goes back to the mid-20th century, with significant milestones marking its evolution. In 1957, Noam Chomsky’s work on “Syntactic Structures” introduced the concept of universal grammar, laying a foundational framework for understanding the structure of language that would later influence NLP development. This was around the same time as the Georgetown experiment in 1954, which showcased the potential for machine translation by automatically translating more than sixty Russian sentences into English, setting an optimistic but ultimately premature expectation for the field’s development.
The 1960s and 1970s saw the development of early NLP systems such as SHRDLU, which operated in restricted environments, and conceptual models for natural language understanding introduced by Roger Schank and others. This period was marked by the use of hand-written rules for language processing.
A significant shift occurred in the late 1980s with the advent of machine learning (ML) algorithms for language processing, moving away from rule-based systems to statistical models. This shift was driven by increased computational power and a move towards corpus linguistics, which relies on analyzing large datasets of language to learn patterns and make predictions. This era saw the development of systems that could take advantage of existing multilingual corpora, significantly advancing the field of machine translation.
The introduction of neural network models in the 1990s and beyond, especially recurrent neural networks (RNNs) and their variant Long Short-Term Memory (LSTM) networks, marked the latest phase in NLP development. These models have significantly improved the ability of machines to process and generate human language, leading to the creation of advanced language models like GPT-3. Such models have demonstrated impressive capabilities in generating human-like text and solving complex language-based tasks, although they do not truly “understand” language in the human sense but rather simulate understanding through sophisticated programming and statistical inference.
Today, NLP and NLU technologies are the basis for a wide array of applications, from voice-activated GPS systems and customer service chatbots to sophisticated language translation programs and virtual assistants such as Siri, Alexa and Google Assistant. These technologies have transformed how humans interact with machines, making it possible to communicate in natural language and have machines interpret, understand, and respond in ways that are increasingly seamless and intuitive.
Related Article: 4 Ways AI, Analytics and Machine Learning Are Improving Customer Service and Support
NLU and NLP in Consumer Insight Gathering
The application of NLU and NLP in analyzing customer feedback, social media conversations, and other forms of unstructured data has become a game-changer for businesses aiming to stay ahead in an increasingly competitive market. These technologies enable companies to sift through vast volumes of data to extract actionable insights, a task that was once daunting and time-consuming. By applying NLU and NLP, businesses can automatically categorize sentiments, identify trending topics, and understand the underlying emotions and intentions in customer communications. This automated analysis provides a comprehensive view of public perception and customer satisfaction, revealing not just what customers are saying, but how they feel about products, services, brands, and their competitors.
Diana Zheng, head of marketing at the Canadian ecommerce shipping company, Stallion Express, told CMSWire that Natural Language Understanding and Natural Language Processing have transformed marketing, advertising, and CX by helping her brand understand the nuances of consumer language. “NLU and NLP allow marketers to craft personalized, impactful messages that build stronger audience relationships,” said Zheng. “By understanding the nuances of human language, marketers have unprecedented opportunities to create compelling stories that resonate with individual preferences.”
The insights gained from NLU and NLP analysis are invaluable for informing product development and innovation. Companies can identify common pain points, unmet needs, and desired features directly from customer feedback, guiding the creation of products that truly resonate with their target audience. This direct line to customer preferences helps ensure that new offerings are not only well-received but also meet the evolving demands of the market.
In addition, NLU and NLP significantly enhance customer service by enabling more efficient and personalized responses. Automated systems can quickly classify inquiries, route them to the appropriate department, and even provide automated responses for common questions, reducing response times and improving customer satisfaction. Understanding the sentiment and urgency of customer communications allows businesses to prioritize issues, responding first to the most critical concerns.
In the realm of targeted marketing strategies, NLU and NLP allow for a level of personalization previously unattainable. By analyzing individual behaviors and preferences, businesses can tailor their messaging and offers to match the unique interests of each customer, increasing the relevance and effectiveness of their marketing efforts. This personalized approach not only enhances customer engagement but also boosts the efficiency of marketing campaigns by ensuring that resources are directed toward the most receptive audiences.
Personalization and Targeted Advertising
NLU and NLP have become pivotal in the creation of personalized marketing messages and content recommendations, driving engagement and conversion by delivering highly relevant and timely content to consumers. These technologies analyze consumer data, including browsing history, purchase behavior, and social media activity, to understand individual preferences and interests. By interpreting the nuances of the language that is used in searches, social interactions, and feedback, NLU and NLP enable marketers to tailor their communications, ensuring that each message resonates personally with its recipient.
Colin Hannan, principal at Proven Partners, an international real estate and resort development consulting firm, reiterated that NLU and NLP are game-changers for his firm — they are now able to generate hyper-personalized content and ads that speak directly to their audience’s desires, gleaned from online behavior and preferences. “We use NLU to analyze customer feedback so we can proactively address concerns and improve CX,” said Hannan.
One notable example of a prominent brand that has leveraged the power of NLU and NLP for personalization is Netflix. The streaming service uses these technologies to analyze viewing habits and reviews, allowing it to recommend shows and movies that match the individual tastes of its users. This not only keeps subscribers engaged but also makes the discovery of new content effortless and enjoyable, contributing to Netflix’s high retention rates.
Amazon’s recommendation system provides another powerful case study. By employing NLP to understand customer reviews and queries, combined with analyzing purchase history, Amazon offers highly personalized product recommendations. This sophisticated approach not only enhances the shopping experience but also significantly increases the likelihood of purchases, demonstrating the direct impact of personalized marketing on conversion rates.
Spotify’s “Discover Weekly” playlist further exemplifies the effective use of NLU and NLP in personalization. By analyzing the songs its users listen to, the lyrics of those songs, and users’ playlist creations, Spotify crafts personalized playlists that introduce users to new music tailored to their individual tastes. This feature has been widely praised for its accuracy and has played a key role in user engagement and satisfaction.
Enhancing Customer Experiences With Conversational AI
The application of NLU and NLP technologies in the development of chatbots and virtual assistants marked a significant leap forward in the realm of customer service and engagement. These sophisticated tools are designed to interpret and respond to user queries in a manner that closely mimics human interaction, thereby providing a seamless and intuitive customer service experience. By using NLU and NLP, chatbots can comprehend the intent and context of customer inquiries, enabling them to deliver accurate and relevant responses, guide users through complex processes, or even escalate issues to human agents when necessary.
One of the key advantages of using NLU and NLP in virtual assistants is their ability to provide round-the-clock support across various channels, including websites, social media, and messaging apps. This ensures that customers can receive immediate assistance at any time, significantly enhancing customer satisfaction and loyalty. Additionally, these AI-driven tools can handle a vast number of queries simultaneously, reducing wait times and freeing up human agents to focus on more complex or sensitive issues.
The sophistication of NLU and NLP technologies also allows chatbots and virtual assistants to personalize interactions based on previous interactions or customer data. This personalization can range from addressing customers by name to providing recommendations based on past purchases or browsing behavior. Such tailored interactions not only improve the customer experience but also help to build a deeper sense of connection and understanding between customers and brands.
“NLU and NLP have had a huge impact on the customer experience,” said Zheng. “NLU allows chatbots and virtual assistants (VAs) to understand user queries and provide more precise and contextually relevant answers. Not only does this make customer interactions more efficient, but it also ensures a smooth and personalized customer journey, increasing brand loyalty.”
Overcoming Language Barriers and Expanding Global Reach
NLU and NLP are instrumental in enabling brands to break down the language barriers that have historically constrained global outreach. Through the use of these technologies, businesses can now communicate with a global audience in their native languages, ensuring that marketing messages are not only understood but also resonate culturally with diverse consumer bases. NLU and NLP facilitate the automatic translation of content, from websites to social media posts, enabling brands to maintain a consistent voice across different languages and regions. This significantly broadens the potential customer base, making products and services accessible to a wider audience.
However, the challenge in translating content is not just linguistic but also cultural. Language is deeply intertwined with culture, and direct translations often fail to convey the intended meaning, especially when idiomatic expressions or culturally specific references are involved. NLU and NLP technologies address these challenges by going beyond mere word-for-word translation. They analyze the context and cultural nuances of language to provide translations that are both linguistically accurate and culturally appropriate. By understanding the intent behind words and phrases, these technologies can adapt content to reflect local idioms, customs, and preferences, thus avoiding potential misunderstandings or cultural insensitivities.
Lyn Collanto, marketing specialist at KBA Web, an Australian SEO agency, told CMSWire that while translating complex language intricacies is still improving, AI’s progress is bringing more humanity into companies’ ability to grasp customers clearly and respond in relevant ways that people appreciate,” said Collanto. “The possibilities of that leading to deeper customer relationships feel exciting as this all advances.”
Despite these advancements, certain challenges persist. The subtleties of humor, sarcasm, and idiomatic expressions can still be difficult for NLU and NLP to accurately interpret and translate. To overcome these hurdles, brands often supplement AI-driven translations with human oversight. Linguistic experts review and refine machine-generated translations to ensure they align with cultural norms and linguistic nuances. This hybrid approach leverages the efficiency and scalability of NLU and NLP while ensuring the authenticity and cultural sensitivity of the content.
Other issues, such as data privacy, remain challenging to alleviate. “Data privacy concerns are the hardest to navigate,” said Hannan. “Most of the time we target high-net-worth individuals (HWNI) and it is a challenge to find a way to reach out to them without violating their privacy. With HNWI, it’s always best to be prepared to adapt in accordance with their wishes. And above all, discretion is everything.”
Final Thoughts
NLP and NLU are transforming marketing and customer experience by enabling levels of consumer insights and hyper-personalization that were previously unheard of. From decoding feedback and social media conversations to powering multilanguage engagement, these technologies are driving connections through cultural nuance and relevance. Where meaningful relationships were once constrained by human limitations, NLP and NLU liberate authentic interactions, heralding a new era for brands and consumers alike.
[ad_2]
Source link